How AI Thinks: A Surprisingly Simple Explanation
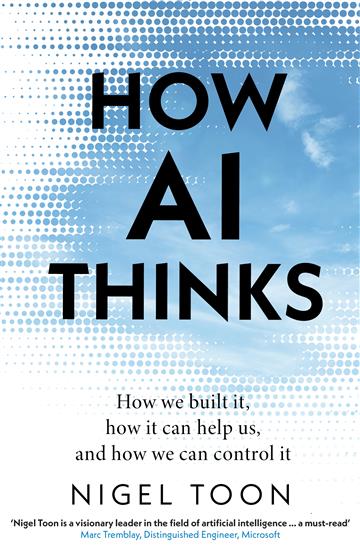
Table of Contents
The Foundation: Data and Algorithms
At the heart of every AI system lies a powerful combination: data and algorithms. Data is the fuel, and algorithms are the engine. Machine learning, a core component of AI, relies heavily on vast quantities of training data. This data, often referred to as big data or simply datasets, provides the raw material for AI to learn from.
- AI systems learn from massive datasets, sometimes containing billions of data points.
- The quality and quantity of data directly impact the performance of the AI system. Garbage in, garbage out, as the saying goes. Inaccurate or biased data will lead to inaccurate or biased results.
- Sophisticated algorithms process this data, searching for patterns, relationships, and insights that humans might miss.
Several types of algorithms drive these processes:
- Supervised learning: The algorithm learns from labeled data, where each data point is tagged with the correct answer. Think of teaching a dog new tricks – you show it the desired behavior and reward it for getting it right.
- Unsupervised learning: The algorithm finds patterns in unlabeled data without any pre-defined answers. This is more like exploring a new city without a map – you discover things as you go.
- Reinforcement learning: The algorithm learns through trial and error, receiving rewards for correct actions and penalties for incorrect ones. This is akin to training a robot to navigate a maze – it learns by trying different paths and getting feedback on its success.
Pattern Recognition: The Heart of AI Thinking
Once an AI system has ingested data, the next step is pattern recognition. This is where the magic truly happens. AI uses sophisticated statistical analysis and techniques like pattern matching and feature extraction to identify hidden correlations and relationships within the data. This is the foundation of predictive modeling, allowing AI to forecast future outcomes based on past trends.
- AI uses statistical methods to find correlations and relationships within the data, often far beyond human capacity.
- This pattern recognition is what allows AI to make predictions and decisions.
- Examples of this are everywhere: image recognition in self-driving cars, spam filtering in your email, and personalized recommendations on streaming services.
However, it's crucial to acknowledge the limitations of pattern recognition. If the training data contains biases, the AI system will likely inherit and amplify those biases. This is a significant area of ongoing research and development within the field of AI ethics.
Decision-Making Processes in AI
Based on the patterns it identifies, AI then makes decisions. This decision-making process is often complex, but it boils down to assigning probabilities to different outcomes. Tools like decision trees can visually represent this process, showing the different pathways and potential results.
- AI uses algorithms to assign probabilities to different outcomes, allowing it to choose the most likely or desirable course of action.
- Decision trees are a useful way to visualize the decision-making process, making it easier to understand how the AI arrives at its conclusions.
- AI systems continuously refine their decision-making based on new data and feedback, improving their accuracy and efficiency over time. This is a key aspect of machine learning's iterative nature.
Different approaches exist for AI decision-making. Some prioritize speed and efficiency, while others prioritize accuracy and robustness. The optimal approach often depends on the specific application and its constraints.
Beyond the Basics: More Complex AI "Thinking"
While the fundamentals of data, algorithms, and pattern recognition explain much of how AI thinks, more advanced techniques add layers of complexity. Deep learning, a subset of machine learning, employs neural networks – complex structures loosely modeled after the human brain – to analyze data in incredibly intricate ways. Natural Language Processing (NLP) allows AI to understand and process human language, facilitating communication and interaction.
- Deep learning allows AI to learn far more complex patterns from vast amounts of data than simpler algorithms.
- Neural networks, with their interconnected layers of nodes, can handle highly complex relationships and datasets.
- NLP enables AI to understand and process human language, opening up new possibilities for communication and interaction with AI systems.
These advanced techniques build upon the simpler methods already discussed, allowing AI to tackle increasingly complex problems.
Understanding How AI Thinks
In conclusion, how AI thinks boils down to algorithms processing large datasets to identify patterns and make decisions. It's not human-like thinking, but a powerful and increasingly sophisticated form of computation. The core principles – data, algorithms, and pattern recognition – are surprisingly simple, yet their combination enables AI to perform remarkable feats. Want to dive deeper into how AI works? Explore these resources on machine learning, deep learning, and various AI applications! (link to relevant resources)
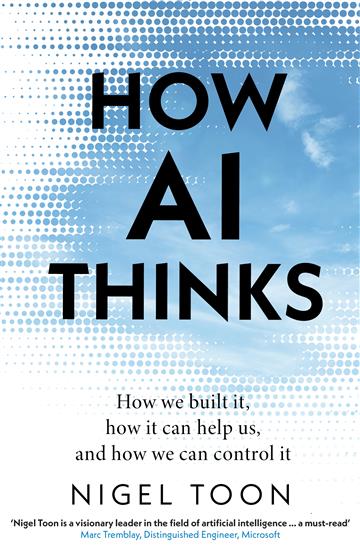
Featured Posts
-
Convicted Cardinal Claims Entitlement To Vote For Next Pope
Apr 29, 2025 -
Capital Summertime Ball 2025 Tickets Everything You Need To Know
Apr 29, 2025 -
Will Minnesota Film Tax Credits Attract More Productions
Apr 29, 2025 -
Family Pleads For Help Locating Missing British Paralympian In Las Vegas
Apr 29, 2025 -
British Paralympian Sam Ruddock Missing Las Vegas Police Investigation
Apr 29, 2025