Understanding AI's "Thought" Processes: A Look At Current Capabilities
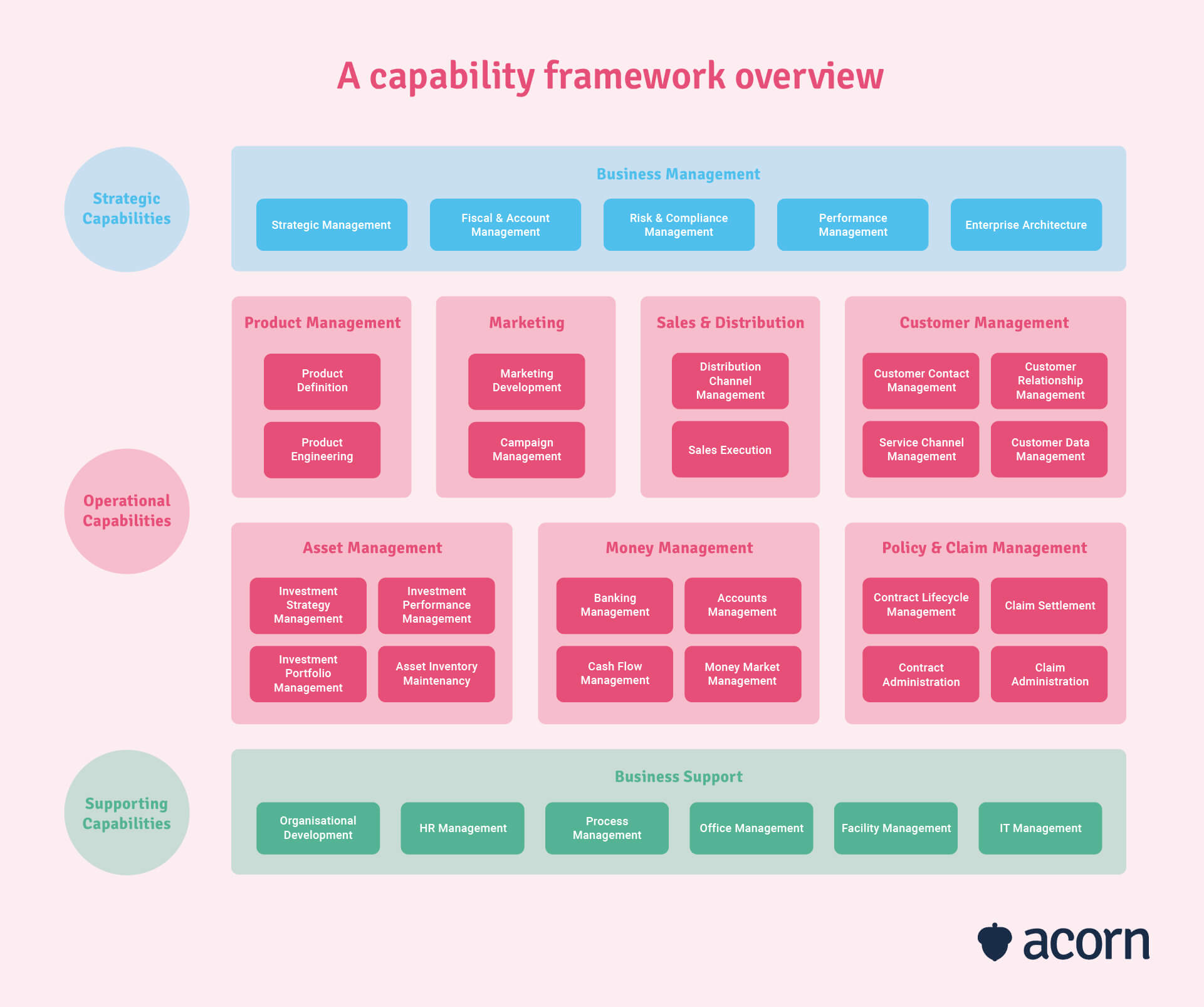
Table of Contents
The Mechanics of AI Decision-Making
AI's decision-making isn't based on consciousness or subjective experience, but rather on sophisticated algorithms that allow it to learn from data and make predictions. Let's delve into the core mechanisms:
Machine Learning Algorithms
Machine learning algorithms are the backbone of many AI systems. These algorithms allow computers to learn from data without explicit programming. Different types of machine learning algorithms exist, each suited to various tasks:
- Supervised Learning: The algorithm learns from labeled data, where inputs are paired with desired outputs. Examples include:
- Linear Regression: Predicting a continuous value (e.g., house price based on size).
- Decision Trees: Classifying data into categories (e.g., spam detection).
- Unsupervised Learning: The algorithm learns from unlabeled data, identifying patterns and structures. Examples include:
- Clustering: Grouping similar data points together (e.g., customer segmentation).
- Dimensionality Reduction: Reducing the number of variables while preserving important information.
- Reinforcement Learning: The algorithm learns through trial and error, receiving rewards or penalties for its actions (e.g., training a robot to navigate a maze).
The quality and quantity of training data significantly impact an AI's performance. Biased or incomplete data can lead to inaccurate or unfair outcomes.
Neural Networks and Deep Learning
Neural networks are a type of machine learning algorithm inspired by the structure and function of the human brain. They consist of interconnected nodes (neurons) organized in layers. Deep learning uses neural networks with multiple layers ("deep" networks) to process complex information.
- Layers, Nodes, Weights, and Biases: Information flows through the network, with each connection having a weight that determines its influence. Biases adjust the activation threshold of each neuron. Through a process called backpropagation, the network adjusts its weights and biases to improve its performance.
- Applications of Deep Learning: Deep learning powers many advanced AI applications, including:
- Image Recognition: Identifying objects, faces, and scenes in images.
- Natural Language Processing (NLP): Understanding and generating human language (e.g., chatbots, machine translation).
Limitations of Current AI "Thought"
While impressive, current AI systems have significant limitations compared to human intelligence.
Lack of True Understanding
AI excels at pattern recognition, but this doesn't equate to genuine comprehension. AI systems lack consciousness, subjective experience, and the ability to truly understand the meaning behind the data they process. This can lead to unexpected outcomes:
- Illogical Decisions: An AI might make seemingly illogical decisions due to biases in its training data or a lack of contextual understanding. For example, an AI trained primarily on images of white cats might struggle to recognize black cats.
- Overfitting: The AI might perform well on training data but poorly on unseen data, failing to generalize its learning.
Explainability and Transparency ("The Black Box" Problem)
Many AI systems, particularly deep learning models, are often described as "black boxes." It can be difficult to understand precisely how they arrive at their conclusions. This lack of transparency poses challenges:
- Debugging and Improvement: Understanding the decision-making process is crucial for identifying and correcting errors.
- Ethical Implications: Opaque AI systems can lead to unfair or discriminatory outcomes, especially in sensitive areas like loan applications or criminal justice. Explainable AI (XAI) research aims to address this issue.
Exploring the Future of AI Cognition
Despite current limitations, ongoing research strives to enhance AI's cognitive capabilities.
Advances in AI Research
Several promising areas are pushing the boundaries of AI cognition:
- Neuro-symbolic AI: Combining neural networks with symbolic reasoning to enhance AI's ability to reason and explain its actions.
- Reinforcement Learning Advancements: Developing more efficient and robust reinforcement learning algorithms for complex tasks.
- Explainable AI (XAI): Creating AI systems that can explain their decision-making processes in a transparent and understandable way.
The Ethical Considerations of Advanced AI
As AI systems become more sophisticated, ethical considerations become paramount:
- Bias and Fairness: Mitigating biases in AI systems to ensure fair and equitable outcomes for all.
- Job Displacement: Addressing potential job displacement caused by automation and AI.
- Responsible AI Development: Establishing ethical guidelines and regulations to govern the development and deployment of AI.
Conclusion
Understanding AI's thought processes reveals a fascinating blend of sophisticated algorithms and significant limitations. AI's decision-making relies on powerful machine learning and deep learning techniques, but current systems lack true understanding and raise crucial ethical concerns. The future of AI cognition hinges on addressing these challenges through ongoing research in areas like explainable AI and responsible AI development. Continue your exploration of AI's thought processes by visiting [link to related resource]. Understanding AI is crucial for navigating the future.
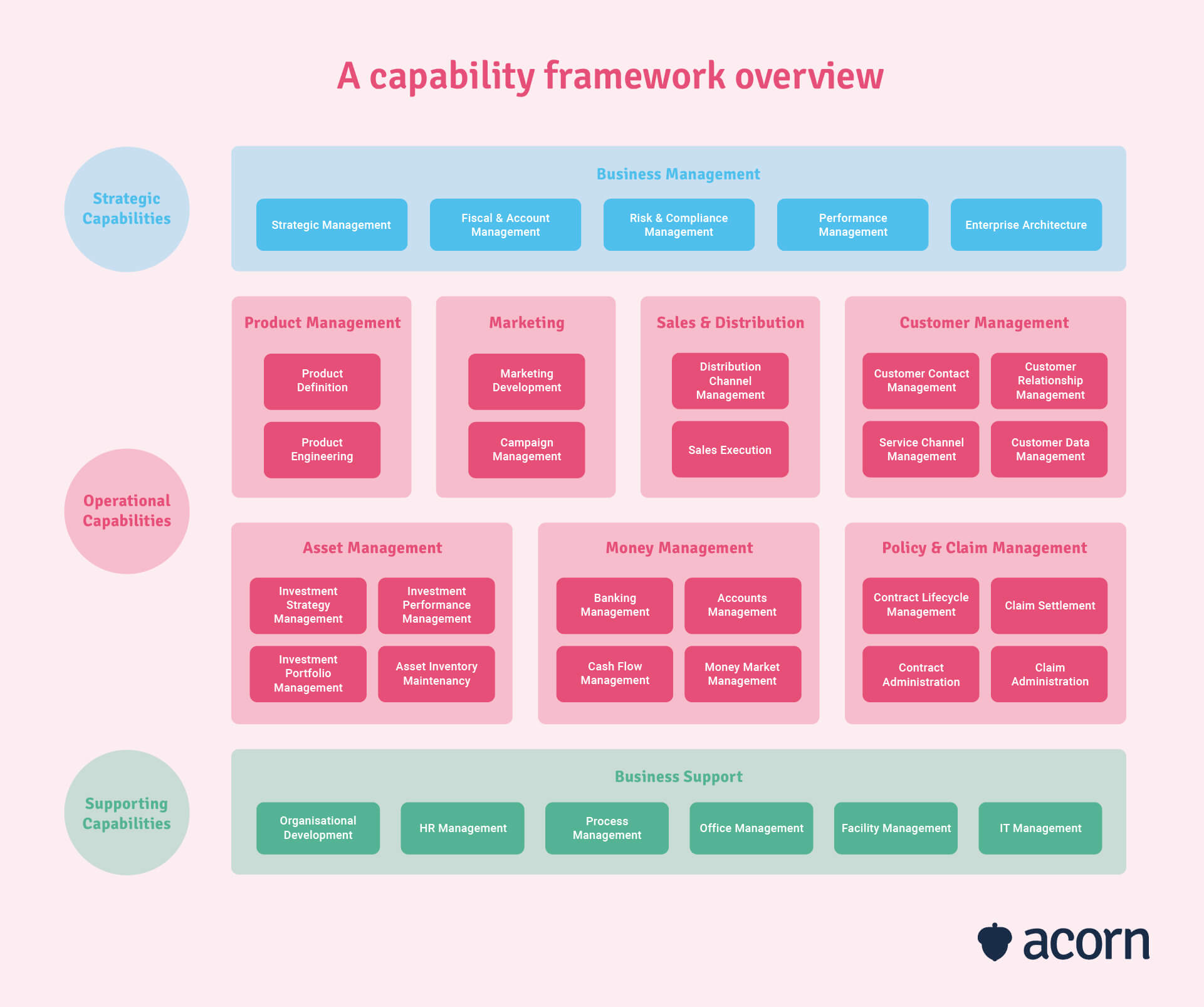
Featured Posts
-
The Thriving Venture Capital Secondary Market Trends And Analysis
Apr 29, 2025 -
Cancer Drug Setback Akeso Stock Plummets On Disappointing Trial Results
Apr 29, 2025 -
March 15 2025 Nyt Spelling Bee Clues Answers And Pangram
Apr 29, 2025 -
The Long Term Impact Of Trumps China Tariffs On Us Consumers And Businesses
Apr 29, 2025 -
Cnn Alan Cumming Recalls Favorite Childhood Activity In Scotland
Apr 29, 2025
Latest Posts
-
Novak Djokovics Upset Loss To Alejandro Tabilo At Monte Carlo Masters 2025
Apr 29, 2025 -
Ramiro Helmeyer A Commitment To Fc Barcelonas Glory
Apr 29, 2025 -
Wnba Bound Diamond Johnson Of Norfolk State Heads To Minnesota Lynx Camp
Apr 29, 2025 -
Justin Herbert Chargers 2025 Season Opener In Brazil Confirmed
Apr 29, 2025 -
Solve The Nyt Spelling Bee Answers And Pangram For April 27 2025
Apr 29, 2025