Outdated Business Applications: A Barrier To Successful AI Adoption
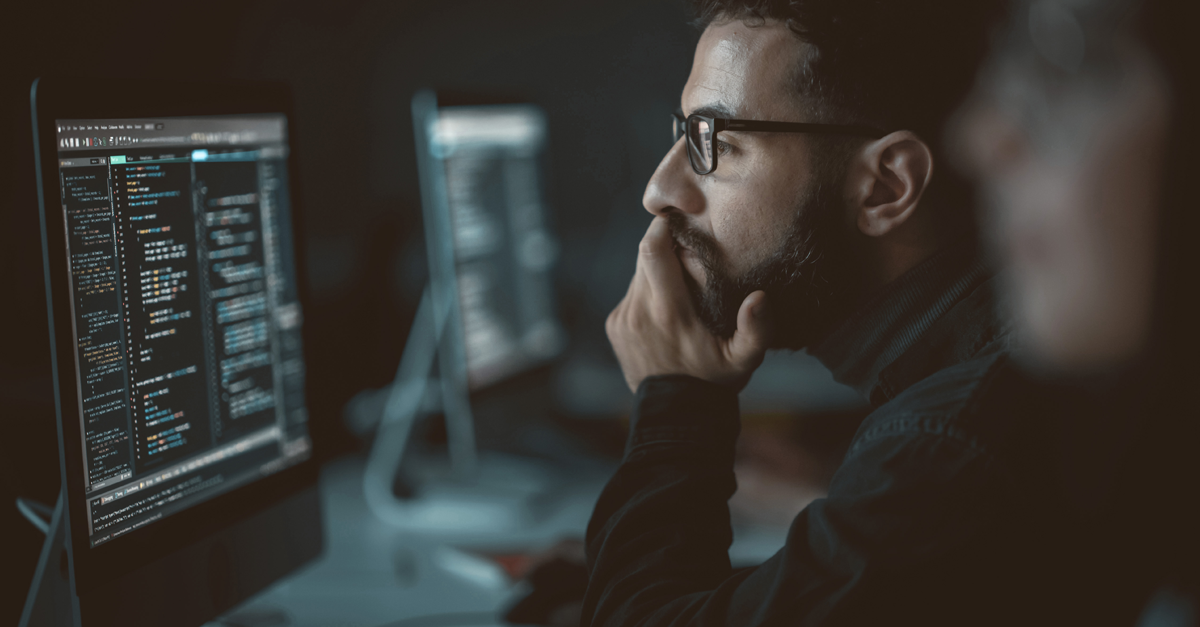
Table of Contents
Data Incompatibility and Integration Challenges
Legacy systems frequently lack the Application Programming Interfaces (APIs) and data structures necessary for seamless AI integration. This incompatibility creates significant roadblocks in the crucial process of feeding data to AI models. Imagine trying to fit a square peg into a round hole—that's the reality many businesses face when attempting to integrate AI with their existing, fragmented systems. Data silos further exacerbate this problem. Information is scattered across disparate systems, making it extremely difficult to consolidate and prepare the data for AI analysis.
- Difficulty in extracting, transforming, and loading (ETL) data for AI models: The process of getting data ready for AI consumption becomes exponentially more complex and time-consuming with incompatible systems.
- Inconsistent data formats hindering accurate AI analysis: Differing data formats across various legacy systems lead to inaccuracies and inconsistencies, ultimately impacting the reliability of AI predictions.
- High costs associated with data migration and cleansing: The task of migrating data from outdated systems and cleaning it to meet AI standards can be an expensive and resource-intensive undertaking.
- Risk of inaccurate AI predictions due to flawed or incomplete data: Using faulty or incomplete data will inevitably lead to unreliable AI predictions, undermining the value of the entire AI initiative.
Limited Scalability and Flexibility
Older applications, often built for specific, limited tasks, struggle to handle the increased data volume and processing demands inherent in AI. AI requires agility and adaptability – something legacy systems rarely offer. Trying to force AI into a rigid, inflexible framework is akin to trying to fit a growing plant into a small, inflexible pot. It restricts growth and can ultimately lead to failure.
- System bottlenecks and performance issues when integrating AI components: Legacy systems often lack the processing power and capacity to handle the additional workload imposed by AI, resulting in bottlenecks and slowdowns.
- Inability to scale AI infrastructure to meet future demands: Outmoded systems often make it difficult, if not impossible, to expand AI infrastructure to accommodate growth and evolving needs.
- Difficulty in deploying and managing AI models effectively: Legacy systems might lack the tools and functionalities required for efficient deployment and management of AI models.
- Higher maintenance costs compared to modern, scalable solutions: Maintaining and updating legacy systems is frequently more expensive than employing modern, scalable alternatives.
Security Risks and Compliance Issues
Outdated systems often represent a significant security vulnerability. They may lack up-to-date security protocols, leaving sensitive data exposed to breaches and attacks. This is a critical concern, especially given the sensitive nature of data often used in AI projects. Furthermore, legacy systems may not comply with modern data privacy regulations like GDPR or CCPA, leading to potential legal repercussions.
- Increased risk of data breaches due to outdated security protocols: Older systems are more susceptible to cyberattacks due to vulnerabilities in their security architecture.
- Non-compliance with GDPR, CCPA, and other relevant regulations: Failure to comply with data privacy regulations can result in hefty fines and reputational damage.
- Potential for legal penalties and reputational damage: Data breaches and non-compliance can lead to significant legal and financial penalties, as well as damage to brand reputation.
- Difficulty in ensuring data integrity and trustworthiness: Outdated systems can make it difficult to guarantee the accuracy, completeness, and reliability of data used in AI applications.
Lack of Automation and Efficiency
Manual processes inherent in many outdated systems create bottlenecks that severely hamper AI's potential for automation. This often results in reduced efficiency, wasted resources, and missed opportunities for optimization. Instead of leveraging AI to streamline processes, businesses may find themselves spending valuable time and resources compensating for the shortcomings of their outdated systems.
- Time-consuming manual data entry and processing: Manually entering and processing data is inefficient and prone to errors.
- Reduced operational efficiency due to system limitations: Outdated applications often impose limitations that decrease overall operational efficiency.
- Increased labor costs and reduced employee productivity: The need to work around outdated systems increases labor costs and reduces employee productivity.
- Missed opportunities for automation and process optimization: Legacy systems hinder the ability to fully utilize AI's automation capabilities.
Strategies for Overcoming the Barrier of Outdated Business Applications
Modernizing legacy systems is crucial for successful AI adoption. A phased approach can mitigate disruption and manage costs effectively. Consider these strategies:
- Cloud migration to leverage scalable and secure infrastructure: Migrating to the cloud provides scalable and secure infrastructure vital for AI initiatives.
- Application modernization to improve functionality and compatibility: Modernizing applications ensures compatibility and enhances functionality.
- Phased upgrades to minimize disruption and manage costs: A phased approach allows for incremental upgrades, minimizing disruption and controlling costs.
- Investing in training and development for employees: Training employees on new systems and processes is crucial for a successful transition.
Conclusion
Outdated business applications pose significant challenges to successful AI adoption. From data incompatibility and security risks to limited scalability and a lack of automation, these legacy systems can severely hinder a company's ability to realize the full potential of AI. Addressing these issues through strategic modernization is not merely an option; it's a necessity for maximizing the benefits of AI. Don't let outdated business applications, or outdated software, hinder your AI journey. Take the first step towards successful AI adoption by assessing your current systems and planning for modernization today. Modernizing business applications is an investment in the future, one that will unlock the true power of AI and pave the way for increased efficiency, profitability, and competitive advantage.
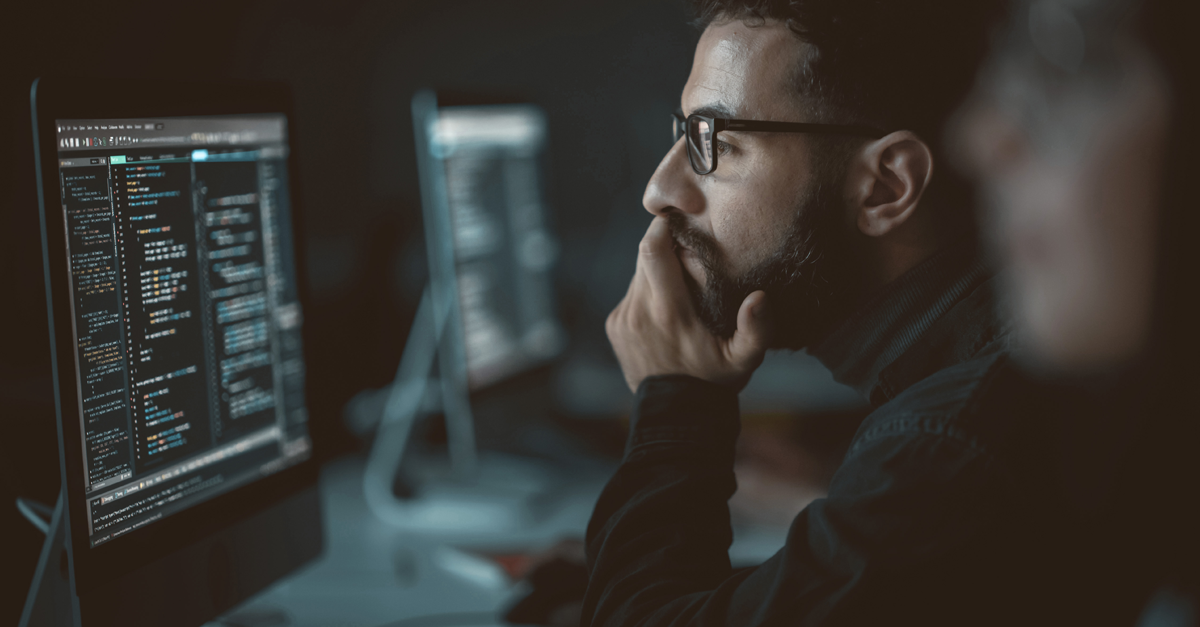
Featured Posts
-
Securing Funding On Dragons Den A Step By Step Approach
May 01, 2025 -
Cleveland Guardians Comeback Win Bibees Resilience Shines
May 01, 2025 -
Fondi 8xmille E Processo Becciu Ultime Notizie Sul Rinvio
May 01, 2025 -
Priscilla Pointer Actress Dies At 100
May 01, 2025 -
Clase Nacional De Boxeo Un Dia Historico En El Zocalo Fotos
May 01, 2025
Latest Posts
-
Geen Gevaar Gaslucht Roden Was Loos Alarm
May 02, 2025 -
Kampen Dagvaardt Enexis Weigering Stroomnetaansluiting
May 02, 2025 -
Gaslucht Roden Loos Alarm
May 02, 2025 -
Energievoorziening Bio Based Basisscholen Een Actueel Probleem
May 02, 2025 -
Juridische Strijd Kampen Eist Stroomnetaansluiting Van Enexis
May 02, 2025