How Outdated Business Apps Obscure Your AI Strategy
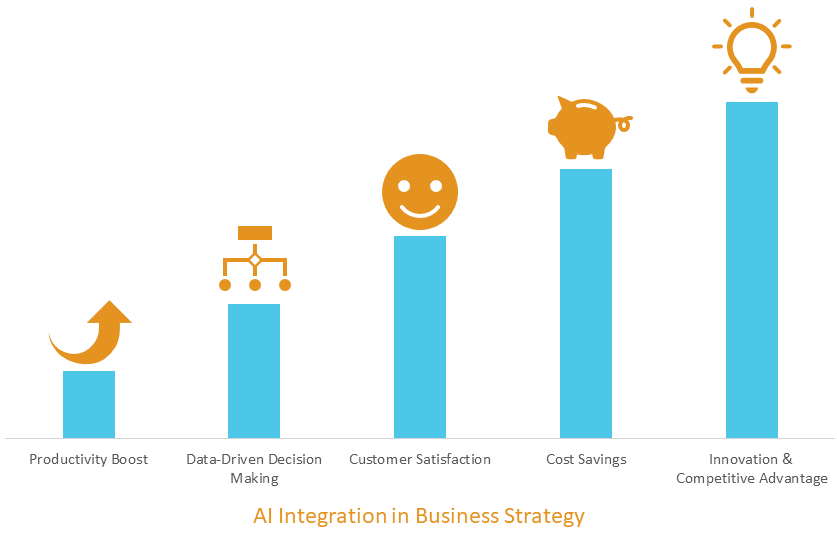
Table of Contents
Data Silos and Integration Challenges
Outdated business apps often create significant data silos, preventing the seamless data flow that is crucial for effective AI implementation. The problem stems from the fact that these systems were not designed with interoperability or data sharing in mind. This results in:
- Difficulty in consolidating data from various sources: Imagine trying to combine sales data from a legacy CRM, marketing data from an outdated email marketing platform, and customer service interactions from a disparate ticketing system. The task becomes nearly insurmountable.
- Inconsistent data formats hindering AI model training: Different applications often use different data formats, making it incredibly challenging to prepare data for AI model training. This necessitates extensive data cleaning and transformation, adding significant time and cost.
- Increased costs and time spent on data cleaning and preparation: The time and resources spent on manually cleaning and preparing data for AI models could be significantly reduced with integrated, modern systems.
- Examples of specific outdated apps causing data silo problems: Legacy CRM systems (like older versions of Salesforce or Microsoft Dynamics), outdated ERP systems (SAP R/3, for instance), and standalone marketing automation tools often contribute significantly to data fragmentation.
Solution: The key to resolving this issue is adopting modern data integration platforms and APIs. These tools facilitate seamless data flow between various applications, enabling the creation of a unified data lake or data warehouse that serves as the foundation for AI initiatives. Consider investing in ETL (Extract, Transform, Load) tools and cloud-based data integration services to streamline this process.
Lack of Scalability and Agility
Outdated apps often lack the flexibility and scalability to handle the increased data volume and processing power demands of AI. This results in several significant challenges:
- Inefficient processing leading to delayed insights: Legacy systems may struggle to process large datasets in a timely manner, delaying valuable insights derived from AI models.
- Difficulty in scaling AI models for increased user base or data volume: As your business grows, your AI models need to scale accordingly. Outdated systems simply cannot keep pace.
- Limited ability to adapt to new AI technologies and algorithms: The rapid evolution of AI requires agile systems that can easily integrate new technologies and algorithms. Legacy systems are often rigid and resistant to change.
- Examples of scalability issues caused by old systems: A monolithic ERP system might struggle to handle the increased transaction volume associated with a rapid business expansion, hindering the ability of AI-powered forecasting models to provide accurate predictions.
Solution: Cloud-based solutions and a microservices architecture provide the necessary scalability and agility. Cloud platforms offer on-demand computing resources, allowing you to easily scale your AI infrastructure as needed. A microservices architecture allows for independent deployment and scaling of individual components, enhancing flexibility and resilience.
Security Risks and Compliance Issues
Outdated business applications often present significant security vulnerabilities, impacting the reliability and data protection capabilities crucial for successful AI implementation. This can lead to:
- Increased risk of data breaches and cyberattacks: Legacy systems often lack the latest security protocols and patches, making them vulnerable to cyberattacks.
- Non-compliance with data privacy regulations (GDPR, CCPA, etc.): Outdated systems may not meet the stringent data privacy requirements mandated by various regulations, leading to hefty fines and reputational damage.
- Difficulty in implementing robust security measures in legacy systems: Integrating modern security tools into legacy systems can be incredibly challenging and costly.
- Examples of security breaches linked to outdated business applications: Numerous high-profile data breaches have been attributed to vulnerabilities in outdated CRM or ERP systems.
The Cost of Inaction
Sticking with outdated apps that hinder AI adoption comes at a significant cost:
- Lost revenue opportunities due to inefficient processes: Delayed insights and lack of automation can lead to lost sales and missed market opportunities.
- Increased operational costs due to manual workarounds: Teams often spend considerable time and resources on manual workarounds to compensate for the limitations of outdated systems.
- Damage to brand reputation due to security breaches or regulatory non-compliance: Security breaches and non-compliance can severely damage a company's reputation, leading to loss of customer trust and significant financial losses.
Solution: Implementing modern security protocols and leveraging cloud-based security solutions are critical for mitigating these risks. Regular security audits, penetration testing, and employee training are also vital.
Choosing the Right Modernization Strategy
Upgrading outdated apps to support AI requires a carefully considered modernization strategy. Several approaches exist:
- Rip and replace: Completely replacing outdated systems with modern alternatives offers a clean slate but can be expensive and time-consuming.
- Integration with modern APIs: Integrating existing systems with modern APIs can provide some level of modernization without a full replacement.
- Modernization through refactoring or cloud migration: Refactoring existing code or migrating to a cloud platform can enhance scalability and agility without a complete replacement.
Considerations: The optimal modernization strategy depends on several factors, including your budget, timeline, business needs, and the complexity of your existing systems. Careful planning and assessment are crucial.
Conclusion: Unlocking AI Potential by Modernizing Your Business Applications
Outdated apps create significant roadblocks to successful AI implementation through data silos, scalability issues, and security risks. Modernizing your business applications is not just a technological upgrade; it's a strategic imperative for unlocking the full potential of AI. Don't let outdated apps obscure your AI strategy. Assess your current application landscape, identify the systems hindering AI adoption, and develop a comprehensive modernization plan. Upgrade your business applications today and unleash the transformative power of AI for your organization. Modernize your tech stack for AI and reap the rewards of improved efficiency, enhanced security, and data-driven decision-making.
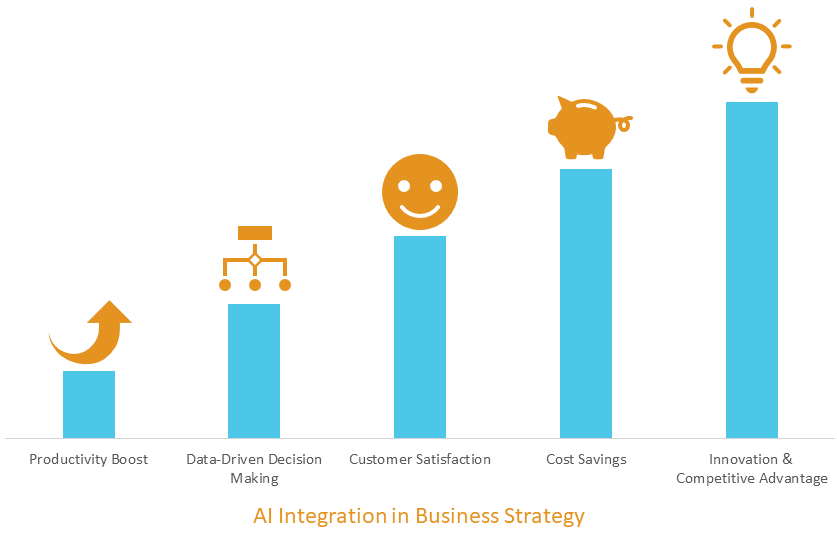
Featured Posts
-
Predicting The Eurovision 2025 Winner Top Contenders Revealed
May 01, 2025 -
Estevao Enjoo E Substituicao Em Partida Do Palmeiras Em Altitude
May 01, 2025 -
Six Nations Rugby Review Frances Winning Campaign Englands Victory Over Wales And Setbacks For Scotland And Ireland
May 01, 2025 -
Ongoing Nuclear Litigation A Comprehensive Overview
May 01, 2025 -
Knattspyrnudagskra T Hrir Leikir I Bestu Deildinni Valur I 2 0 Forystu
May 01, 2025