How Outdated Business Applications Hamper Your AI Strategy
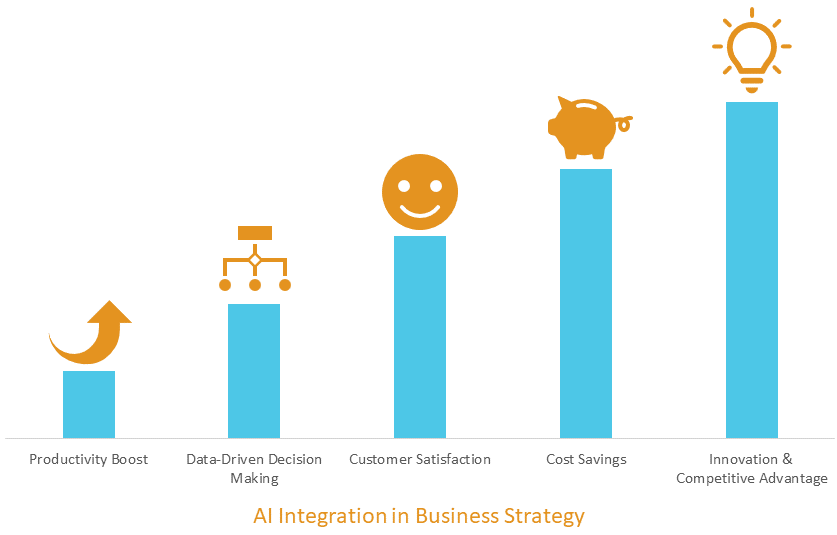
Table of Contents
Data Incompatibility and Integration Challenges
Outdated systems present significant hurdles when it comes to integrating AI. The core problem often lies in the incompatibility of data, stemming from various sources and formats.
Data Silos
Legacy systems frequently create data silos, where data is trapped in isolated systems, making it incredibly difficult to consolidate the information needed for effective AI training.
- Examples of data silos: Customer Relationship Management (CRM) systems, Enterprise Resource Planning (ERP) systems, marketing automation platforms, and separate databases often operate independently, resulting in fragmented data.
- Data cleansing and normalization challenges: Combining data from these disparate sources requires extensive data cleansing and normalization – a complex and time-consuming process. Inconsistent data formats, missing values, and duplicate entries significantly impact the accuracy and reliability of AI models.
- Impact on AI model accuracy: Inaccurate or incomplete data leads to flawed AI models, reducing their predictive power and making them unreliable for decision-making. This directly translates to poor business outcomes.
Data Format Issues
Beyond data silos, the sheer incompatibility of data formats between legacy systems and modern AI platforms poses another significant obstacle.
- Examples of incompatible formats: Outdated systems may use proprietary formats, while AI platforms often rely on standardized formats like CSV, JSON, or Parquet. Converting between these formats can be a major undertaking.
- Challenges in data conversion: Manually converting data formats is not only time-consuming but also prone to errors. Automated conversion tools may be necessary, requiring additional investment and expertise.
- Need for specialized tools and expertise: Successfully navigating these data format issues requires specialized tools and skilled data engineers, adding to the overall cost and complexity of AI implementation.
Limited API Access
Many outdated business applications lack robust Application Programming Interfaces (APIs) or have severely limited API access. This drastically restricts the ability to seamlessly access and integrate data for AI purposes.
- Importance of APIs for seamless data flow: APIs enable automated data transfer between systems, crucial for efficient AI model training and deployment.
- Cost and effort of reverse engineering data access: Without proper APIs, organizations might have to resort to cumbersome and expensive workarounds, such as reverse engineering data access or developing custom connectors.
- Implications for AI development speed: The lack of APIs significantly slows down the AI development process, delaying the realization of business benefits.
Scalability and Performance Limitations
Legacy systems are often ill-equipped to handle the computational demands of modern AI applications.
Infrastructure Constraints
Outdated infrastructure is a significant bottleneck for AI deployment.
- Issues with processing power: AI model training often requires substantial computing power, which legacy systems may lack.
- Memory limitations: Large datasets needed for AI training can exceed the memory capacity of older systems, hindering efficient processing.
- Network bandwidth constraints: Transferring large amounts of data for AI training requires sufficient network bandwidth, which can be a constraint with outdated infrastructure.
- Impact on AI model training time and performance: These infrastructural limitations significantly increase AI model training time and can negatively impact model performance.
Lack of Agility and Flexibility
Adapting legacy systems to accommodate new AI algorithms and technologies is often a significant challenge.
- Challenges in integrating new AI tools: Integrating modern AI tools and libraries with outdated systems can be difficult and require extensive customization.
- Limitations in deploying and scaling AI models: Deploying and scaling AI models efficiently is challenging with inflexible legacy systems.
- Impact on innovation and responsiveness: The lack of agility and flexibility hinders innovation and makes it difficult for businesses to respond quickly to changing market demands.
Security Risks and Compliance Issues
Outdated systems pose significant security and compliance risks that can undermine the entire AI strategy.
Vulnerability to Cyber Threats
Legacy systems often lack the modern security protocols required to protect sensitive data used in AI applications.
- Examples of vulnerabilities: Outdated software versions, lack of security patches, and weak authentication mechanisms create vulnerabilities that cybercriminals can exploit.
- Risk of data breaches: Data breaches can lead to financial losses, reputational damage, and legal liabilities.
- Implications for AI model security and trust: Compromised data can lead to inaccurate or biased AI models, eroding user trust and potentially causing significant harm.
Regulatory Compliance Challenges
Legacy systems might not comply with modern data privacy regulations like GDPR and CCPA.
- Legal and financial risks of non-compliance: Non-compliance can result in hefty fines and legal repercussions.
- Need for data governance and security measures: Implementing robust data governance and security measures is crucial to comply with regulations.
- Implications for AI model deployment: Failure to comply with data privacy regulations can prevent the deployment of AI models that use sensitive data.
The Cost of Inaction
Ignoring the challenges posed by outdated business applications comes at a significant cost.
Lost Opportunities
Ineffective AI implementation directly impacts the bottom line.
- Examples of lost revenue: Missed opportunities for automation, optimization, and improved decision-making directly translate to lost revenue.
- Reduced efficiency: Inefficient processes hampered by outdated systems reduce overall operational efficiency.
- Missed competitive advantages: Competitors leveraging modern technology and AI gain a significant advantage.
Increased Operational Costs
Maintaining and supporting outdated systems incurs ongoing expenses.
- Maintenance costs: Maintaining legacy systems often requires specialized skills and expertise, driving up maintenance costs.
- Technical debt: Accumulated technical debt from years of patching and workarounds increases complexity and costs.
- Decreased productivity: Employees spend more time working around system limitations, reducing overall productivity.
Conclusion
Outdated business applications present significant challenges to successful AI implementation. Data incompatibility, scalability limitations, security risks, and escalating costs are major roadblocks. Don't let outdated business applications hinder your AI strategy. Assess your current systems and invest in modernization to achieve optimal AI implementation and reap the benefits of advanced technology. Embracing digital transformation and modernizing your technology infrastructure is crucial to unlock the full potential of your AI strategy and gain a competitive edge.
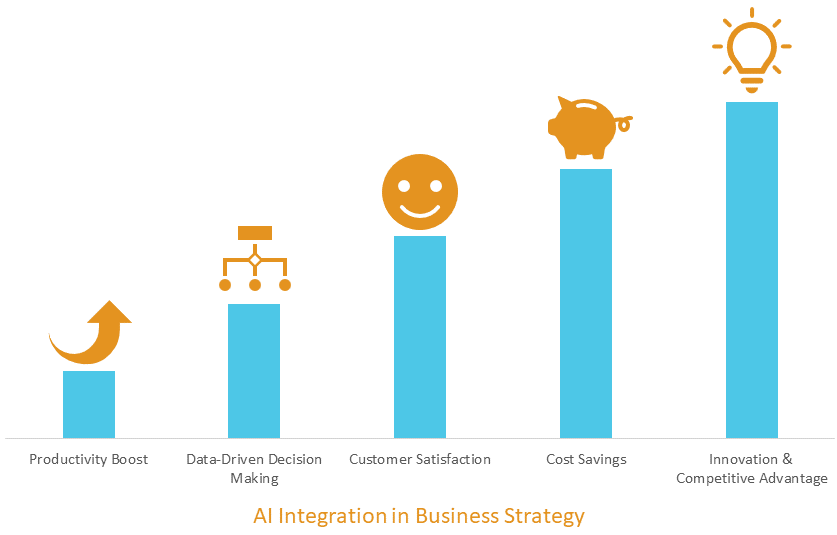
Featured Posts
-
The Intricacies Of Papal Funeral Seating Arrangements
Apr 30, 2025 -
Bestu Deildin I Dag Dagskra Og Urskurdur A Valur Leik
Apr 30, 2025 -
Trail Blazers Play In Push Assessing Their Realistic Chances
Apr 30, 2025 -
Campaigners Deep Concern A Critical Review Of Police Accountability
Apr 30, 2025 -
Eurovision 2025 Song Remember Mondays Capital Breakfast Reveal
Apr 30, 2025
Latest Posts
-
Port Talbot Neighbours Benefit From Michael Sheens 1 Million Debt Payoff
May 01, 2025 -
Leading Cause Of Early Death Doctor Names A Food More Dangerous Than Smoking
May 01, 2025 -
Michael Sheens Generous Act 1 Million Debt Relief For Port Talbot Neighbours
May 01, 2025 -
The Silent Killer Doctor Links This Food To Higher Early Death Rates Than Smoking
May 01, 2025 -
Michael Sheens Port Talbot Home Paying Off Neighbours Debts
May 01, 2025