Google Vs. OpenAI: A Deep Dive Into I/O And Io Technologies
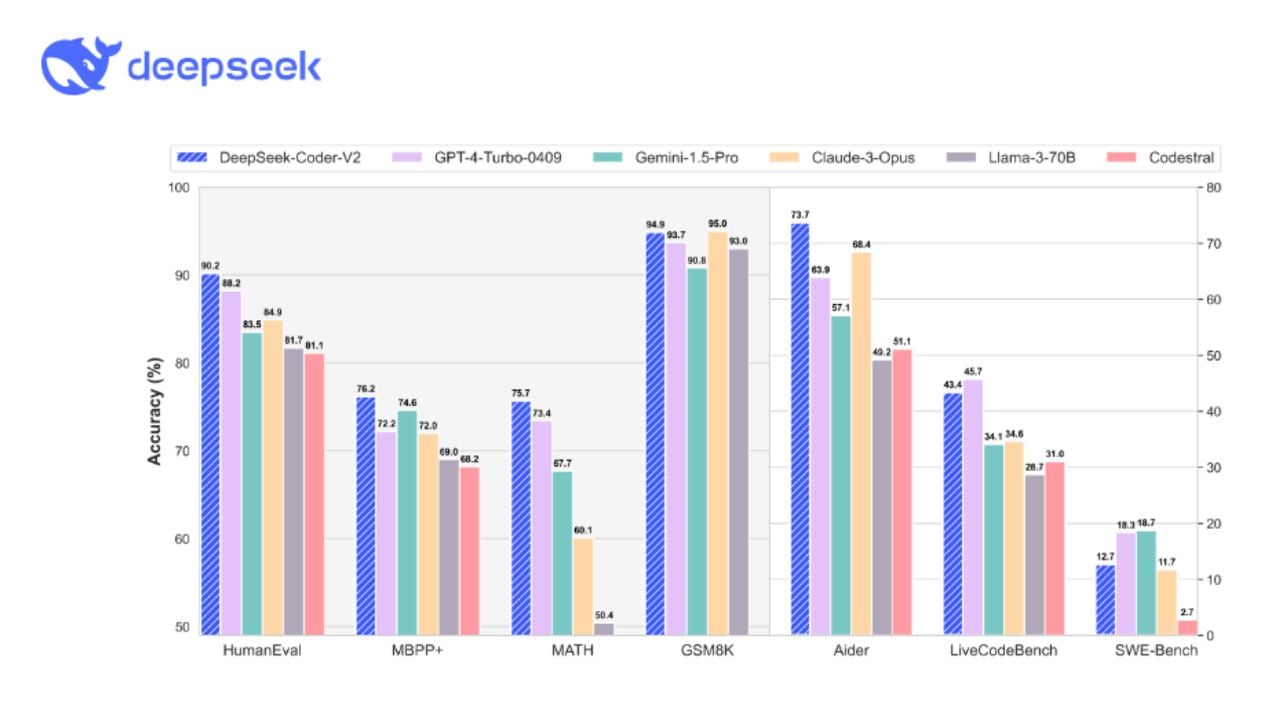
Table of Contents
Google's I/O and io Technologies
Google's approach to I/O is deeply intertwined with its massive infrastructure and commitment to scalability. Their strategies are built to handle the immense data volumes and computational demands of cutting-edge AI.
Google's Emphasis on Scalability and Infrastructure
Google prioritizes large-scale data processing and efficient management of I/O-bound operations. This is facilitated by its extensive cloud infrastructure, Google Cloud Platform (GCP), which offers seamless I/O capabilities. They leverage advanced techniques to optimize I/O performance, including:
- Distributed File Systems: Google File System (GFS) and its successors allow for the efficient storage and retrieval of massive datasets across numerous machines. This is crucial for training and deploying large language models.
- Data Pipelines: Tools like Apache Beam and Cloud Dataflow enable the construction of robust and scalable data pipelines, ensuring smooth data flow for AI model training and inference.
- Container Orchestration: Google Kubernetes Engine (GKE) manages containerized applications, allowing for efficient scaling and resource allocation, which is critical for managing I/O demands during peak usage.
- Scalable Object Storage: Google Cloud Storage provides highly scalable and durable object storage, essential for storing and accessing the vast amounts of data required by AI models.
Google's I/O in AI Models
Google integrates I/O considerations directly into the design of its large language models (LLMs), such as LaMDA and PaLM. This focus on I/O efficiency translates to:
- Faster Training Times: Optimized I/O pipelines significantly reduce the time required to train massive AI models.
- Improved Inference Speeds: Efficient I/O management ensures quick response times when users interact with AI models.
- Specialized Hardware: Google's Tensor Processing Units (TPUs) are custom-designed hardware accelerators that significantly improve the speed of I/O operations, particularly beneficial for large-scale AI model training and inference.
- TensorFlow Data Input Pipelines: TensorFlow, Google's machine learning framework, provides sophisticated data input pipelines designed to maximize I/O efficiency.
OpenAI's I/O and io Technologies
OpenAI takes a different approach, focusing on ease of access and user experience through its APIs. While their underlying infrastructure is undoubtedly powerful, their emphasis lies in providing readily accessible AI capabilities.
OpenAI's Focus on Model Performance and API Accessibility
OpenAI prioritizes ease of access to its models through well-defined APIs. This approach allows developers to readily integrate powerful AI capabilities into their applications. Key aspects include:
- User-Friendly APIs: The OpenAI API provides a streamlined interface for interacting with models like GPT-3 and DALL-E 2.
- Streamlined Input Formats: OpenAI carefully designs input formats to ensure a smooth user experience and minimize potential I/O bottlenecks.
- Model Optimization: OpenAI focuses on optimizing its models to minimize latency and maximize throughput, ensuring responsive performance for users.
OpenAI's I/O Challenges and Innovations
Managing the I/O demands of increasingly complex models presents ongoing challenges for OpenAI. They are continually innovating to address potential bottlenecks:
- Handling Large Datasets: OpenAI is actively researching methods for efficiently handling the massive datasets required to train and improve its models.
- Efficient Data Encoding: Research into efficient data encoding methods is crucial for minimizing the I/O overhead associated with processing large amounts of data.
- Model Parallelism: OpenAI explores model parallelism techniques to distribute the I/O load across multiple machines, improving scalability and responsiveness.
Comparing Google and OpenAI's I/O Approaches
Google and OpenAI represent distinct yet complementary approaches to I/O in AI. Google's emphasis on infrastructure scalability provides unmatched power for large-scale AI deployments. OpenAI's API-centric strategy, however, democratizes access to cutting-edge AI capabilities.
- Key Differences: Google's focus is on massive infrastructure and scalability, while OpenAI prioritizes API accessibility and user-friendliness. This leads to a trade-off: Google's approach offers immense power but requires significant technical expertise, while OpenAI's provides ease of use but may have limitations in scalability for extremely large projects.
Conclusion
This deep dive into Google and OpenAI's I/O and io technologies reveals distinct yet complementary approaches. Google's emphasis on infrastructure scalability offers unparalleled power for large-scale AI deployments, while OpenAI's API-driven model accessibility democratizes access to cutting-edge AI capabilities. Understanding these differences is critical for developers and researchers. Choosing the right platform depends on your specific needs – massive processing power or streamlined API access. Further exploration of both Google and OpenAI's ongoing innovations in I/O and io technologies will be crucial to shaping the future of AI. Continue learning about Google vs. OpenAI’s advancements in I/O and related technologies to stay ahead in this rapidly evolving field.
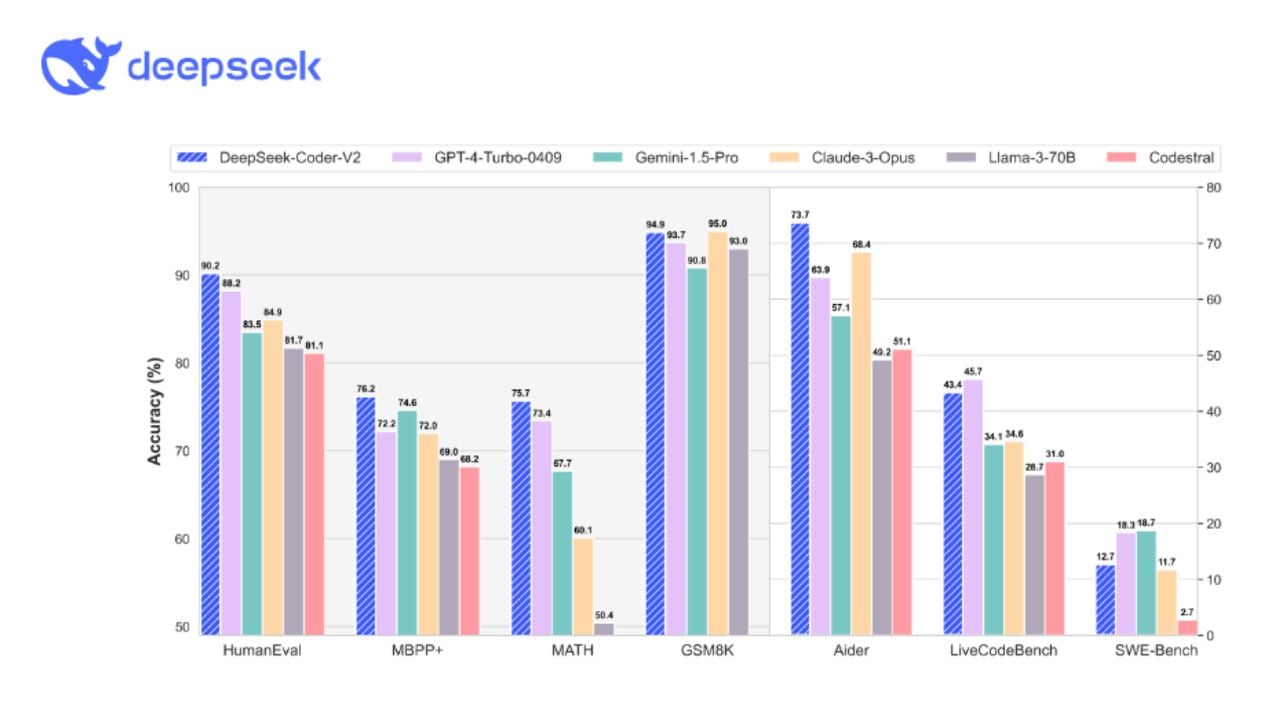
Featured Posts
-
The Sutton Hoo Vessel A Sixth Century Artifact And Its Role In Funerary Practices
May 26, 2025 -
Moto Gp Inggris 2025 Jadwal Terbaru Dan Informasi Balapan
May 26, 2025 -
North Myrtle Beach Water Usage Public Safety Concerns
May 26, 2025 -
Decoding The F1 Drivers Press Conference Key Moments And Takeaways
May 26, 2025 -
La Liga Dramasi Atletico Madrid Hakem Karariyla Espanyol U Gecti
May 26, 2025