D-Wave's Quantum Computing (QBTS) Revolutionizes Drug Discovery With AI
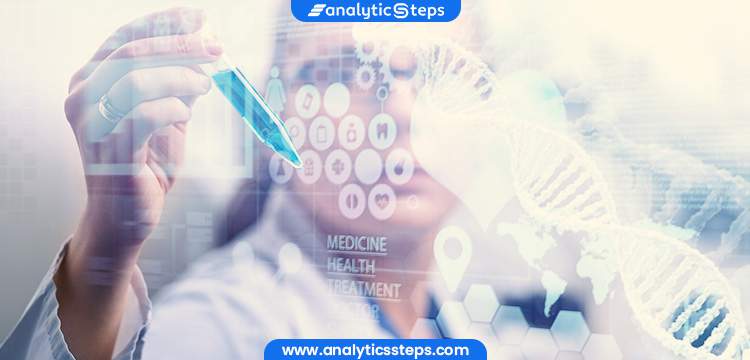
Table of Contents
Accelerating Drug Design with Quantum Annealing (QBTS)
Traditional computing struggles with the complexity of molecular simulations needed for drug design. The sheer number of possible molecular configurations and interactions presents a computational bottleneck, slowing down the drug discovery process considerably. This is where D-Wave's Quantum Annealing (QBTS) offers a game-changing advantage. QBTS excels at tackling optimization problems—finding the best solution among a vast number of possibilities—far more efficiently than classical algorithms.
Overcoming Computational Barriers
- Faster identification of potential drug candidates: QBTS allows researchers to sift through a much larger chemical space, identifying promising drug candidates significantly faster than with traditional methods. This translates to shorter lead times and reduced costs associated with drug development.
- Improved accuracy in predicting drug efficacy and toxicity: By performing more comprehensive simulations, QBTS helps to predict how a drug candidate will interact with its target and potential side effects with greater accuracy. This reduces the risk of failure in later stages of clinical trials.
- Reduced reliance on computationally expensive classical simulations: QBTS can handle problems that are intractable for classical computers, reducing the need for approximations and simplifying the overall drug design workflow.
Optimizing Molecular Interactions
Designing effective drugs relies heavily on understanding and optimizing molecular interactions. Drugs need to bind precisely to their target proteins to elicit the desired therapeutic effect. QBTS is particularly adept at finding the optimal configurations of molecules that maximize this binding affinity.
- Enhanced prediction of protein-ligand interactions: QBTS enables more accurate predictions of how drug molecules (ligands) will interact with their target proteins, leading to more effective drug design.
- More efficient identification of lead compounds with desired properties: By rapidly exploring a wider range of molecular structures, QBTS significantly accelerates the identification of lead compounds that possess the desired pharmacological properties.
- Improved design of drug delivery systems: QBTS can also be applied to optimize the design of drug delivery systems, ensuring that the drug reaches its target effectively and efficiently.
AI's Synergistic Role in Drug Discovery with QBTS
The power of D-Wave's QBTS is amplified when combined with the capabilities of artificial intelligence. AI and quantum computing work synergistically, each leveraging the strengths of the other to create a transformative approach to drug discovery.
Data Analysis and Pattern Recognition
AI algorithms excel at analyzing vast datasets of molecular structures, biological information, and clinical trial data. They can identify subtle patterns and relationships that might be missed by human researchers, generating hypotheses that can then be rigorously tested using QBTS.
- Faster processing of large biological datasets: AI algorithms can quickly process and analyze massive datasets, extracting valuable insights that inform the drug design process.
- Improved identification of relevant biomarkers and drug targets: AI can help identify potential drug targets and biomarkers associated with specific diseases, focusing research efforts on the most promising avenues.
- Enhanced predictive models for drug response and efficacy: AI can develop predictive models that estimate the efficacy and safety of drug candidates, reducing the need for extensive and expensive clinical trials.
AI-Driven Optimization of QBTS Algorithms
AI can also be used to optimize the parameters and performance of the QBTS system itself, making it even more efficient and accurate. This feedback loop between AI and QBTS creates a self-improving system that continuously refines its ability to solve complex drug design problems.
- Improved accuracy of quantum annealing solutions: AI can help to reduce errors and improve the accuracy of solutions generated by QBTS.
- Reduced runtime for complex simulations: AI-driven optimization can significantly decrease the time required to perform complex molecular simulations.
- More effective use of quantum resources: AI can help to optimize the allocation of quantum resources, ensuring that QBTS is used as efficiently as possible.
Real-World Applications and Case Studies of D-Wave's QBTS in Drug Discovery
While specific details of ongoing collaborations are often confidential due to competitive pressures, the potential applications of D-Wave's QBTS in drug discovery are immense.
Examples of Successful Implementations
While detailed case studies might not be publicly available in their entirety due to commercial sensitivities, D-Wave actively collaborates with pharmaceutical companies and research institutions on various projects. These projects explore diverse areas like optimizing drug candidates for specific diseases and designing more effective drug delivery systems. Success is measured through improvements in prediction accuracy, reduction in development time, and cost savings.
Addressing Current Challenges and Future Directions
Despite the significant potential, challenges remain. Noise and errors inherent in current quantum computers need to be addressed to enhance the accuracy of QBTS simulations. Scaling up the system to handle even larger and more complex problems is also crucial for maximizing its impact on drug discovery.
- Addressing noise and error in quantum computations: Ongoing research focuses on improving the stability and reducing noise levels in QBTS systems.
- Scaling up the system to handle even more complex problems: Future generations of QBTS systems will need to be significantly larger to address the complexity of many real-world drug design problems.
- Developing more sophisticated AI algorithms for integration with QBTS: Advancements in AI algorithms are needed to fully leverage the capabilities of QBTS and unlock its full potential.
Conclusion
D-Wave's QBTS, combined with AI, is fundamentally changing the landscape of drug discovery. This powerful combination tackles complex computational challenges, leading to faster drug development, increased accuracy, and potentially life-saving breakthroughs. The future of medicine is being shaped by this innovative approach. To learn more about how D-Wave's quantum computing (QBTS) is revolutionizing drug discovery, explore the latest research and advancements on the D-Wave website. Embrace the future of pharmaceutical innovation with D-Wave's quantum computing (QBTS).
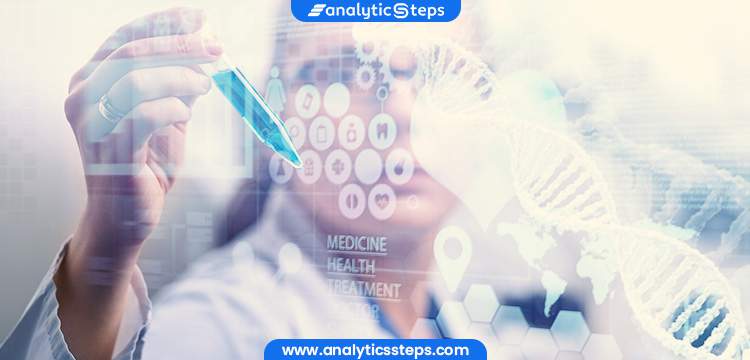
Featured Posts
-
Salaries De La Gaite Lyrique Quittent Les Lieux Securite Assuree Par La Mairie
May 20, 2025 -
Alito And Roberts Two Decades On The Supreme Court Bench
May 20, 2025 -
Aldhkae Alastnaey Yueyd Ihyae Qss Ajatha Krysty Drast Halt
May 20, 2025 -
Continuing Tariff Uncertainty An Fp Video Analysis Of Domestic And International Impacts
May 20, 2025 -
Usmc Tomahawk Missile Launch Army Explores Drone Truck Technology
May 20, 2025
Latest Posts
-
Uspesny Tim Home Office Kancelaria Alebo Kombinacia Obidvoch
May 20, 2025 -
Home Office Alebo Kancelaria Rozhodovanie Na Zaklade Potrieb Zamestnancov A Manazerov
May 20, 2025 -
Vyber Idealneho Pracovneho Prostriedku Home Office Vs Kancelaria
May 20, 2025 -
How To Build A Billionaire Boys Empire Strategies For Young Entrepreneurs
May 20, 2025 -
Preco 79 Manazerov Preferuje Osobny Kontakt Home Office Vs Klasicka Kancelaria
May 20, 2025