Building Trust: The Reliability Of A Robust Poll Data System
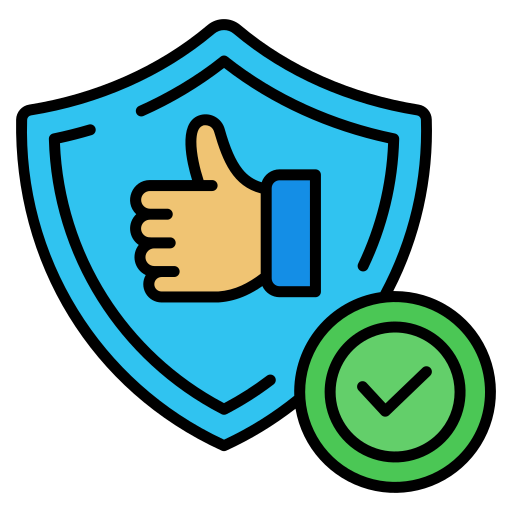
Table of Contents
Data Collection Methodology: The Foundation of Reliable Polls
The foundation of any trustworthy poll data system lies in its data collection methodology. A flawed approach at this stage will inevitably lead to unreliable results, regardless of how sophisticated the subsequent analysis. This section focuses on key aspects ensuring robust and representative data collection.
Sampling Techniques: The Cornerstone of Representativeness
Choosing the right sampling technique is paramount for minimizing bias and ensuring your poll accurately reflects the target population. Poor sampling can lead to drastically skewed results, rendering your poll data useless.
- Random Sampling: Every member of the population has an equal chance of being selected. This method is ideal for minimizing bias but can be challenging to implement for large and dispersed populations.
- Stratified Sampling: The population is divided into subgroups (strata) based on relevant characteristics (e.g., age, gender, location), and random samples are drawn from each stratum. This ensures representation from all subgroups, even if some are smaller.
- Cluster Sampling: The population is divided into clusters (e.g., geographic areas), and a random sample of clusters is selected. All members within the selected clusters are then surveyed. This is cost-effective for large geographical areas but may introduce cluster-specific biases.
For example, relying solely on online surveys might exclude individuals without internet access, leading to a biased sample and inaccurate representation of the overall population. Similarly, using a convenience sample (e.g., surveying only your friends) will severely limit the generalizability of your findings.
Data Collection Methods: Choosing the Right Approach
The method you choose to collect your data significantly impacts its reliability. Each approach comes with its own set of strengths and weaknesses, and potential biases.
- Online Surveys: Cost-effective and convenient for large-scale data collection, but susceptible to self-selection bias (only certain types of people participate) and issues with data quality control.
- Phone Interviews: Allow for clarification and probing of responses, but suffer from lower response rates and potential interviewer bias.
- In-person Interviews: Offer the highest level of control and engagement, but are expensive and time-consuming.
Best practices for minimizing bias include using neutral language, ensuring anonymity, and implementing rigorous quality control checks.
Survey Design and Question Wording: Clarity is Key
The clarity and neutrality of your survey questions are crucial for obtaining reliable data. Ambiguous or leading questions can significantly skew results.
- Avoid leading questions: Instead of asking "Don't you agree that...?" try "What is your opinion on...?"
- Use clear and concise language: Avoid jargon or technical terms that respondents might not understand.
- Keep questions neutral: Avoid questions that express a particular viewpoint or encourage a specific response.
For instance, asking "Do you agree that the government is wasting taxpayer money?" is a leading question, while "What is your opinion on government spending?" is more neutral.
Data Processing and Analysis: Ensuring Data Integrity
Accurate data processing and analysis are essential for transforming raw survey responses into meaningful insights. This involves several crucial steps to maintain data integrity.
Data Cleaning and Validation: Refining the Raw Data
Data cleaning is the process of identifying and addressing errors, inconsistencies, and missing data in your dataset. This is crucial for ensuring the accuracy of your analysis.
- Missing Data: Strategies include imputation (estimating missing values) or listwise deletion (excluding cases with missing data).
- Outliers: Extreme values that might distort the results can be identified and handled using various techniques, such as winsorizing or trimming.
- Inconsistencies: Errors or contradictions in responses can be identified and corrected through careful data review and validation.
Ignoring these issues can lead to inaccurate conclusions and undermine the trustworthiness of your poll data.
Statistical Analysis: Choosing the Right Methods
Appropriate statistical methods are vital for interpreting poll data correctly. This includes considering sample size, margin of error, and confidence intervals.
- Sample Size: A larger sample size generally leads to more precise estimates and smaller margins of error.
- Margin of Error: This quantifies the uncertainty associated with your poll estimates.
- Confidence Intervals: These provide a range of values within which the true population parameter is likely to fall.
Transparency in reporting the statistical methods employed is crucial for building trust in the results.
Data Security and Privacy: Protecting Respondent Information
Protecting respondent data is paramount. Robust data security protocols are crucial for maintaining data integrity and ensuring compliance with regulations such as GDPR and CCPA.
- Anonymization: Removing identifying information from the data protects respondent privacy.
- Data Encryption: Protecting data using encryption safeguards against unauthorized access.
- Secure Data Storage: Using secure servers and access controls is critical for preventing data breaches.
Transparency and Accountability: Building Public Trust
Transparency and accountability are essential for building public trust in poll data. This includes openly documenting the entire process and clearly reporting the results.
Methodological Transparency: Openness Builds Confidence
Openly documenting the entire poll data collection and analysis process is essential. Publishing detailed methodology reports allows for scrutiny and enhances the credibility of your poll.
- Detailed Methodology Reports: These reports should include information about sampling techniques, data collection methods, data cleaning procedures, and statistical analyses.
- Peer Review and Independent Audits: Subjecting your methodology to peer review and independent audits adds an extra layer of validation and builds trust.
Result Reporting and Interpretation: Accuracy and Context are Crucial
Accurate and unbiased reporting of poll results is crucial. This includes acknowledging limitations and potential biases, providing context, and avoiding misleading interpretations.
- Clear and Concise Reporting: Present results in an easily understandable format, avoiding technical jargon.
- Contextualization: Provide sufficient context to help readers interpret the results accurately.
- Acknowledging Limitations: Openly discuss the limitations of the study, including potential biases and sources of error.
Conclusion: Building a Reliable Poll Data System
A robust poll data system requires meticulous attention to detail at every stage, from the design of the survey instrument to the reporting of results. Transparency, rigorous statistical analysis, and robust data security protocols are crucial components for building trust and ensuring reliable results. Employing best practices at each stage guarantees accurate and meaningful insights, ultimately leading to better decision-making. Invest in building a reliable poll data system to ensure the accuracy and trustworthiness of your survey results. Contact us today to learn more about how to improve the reliability of your poll data system and gain valuable, actionable insights.
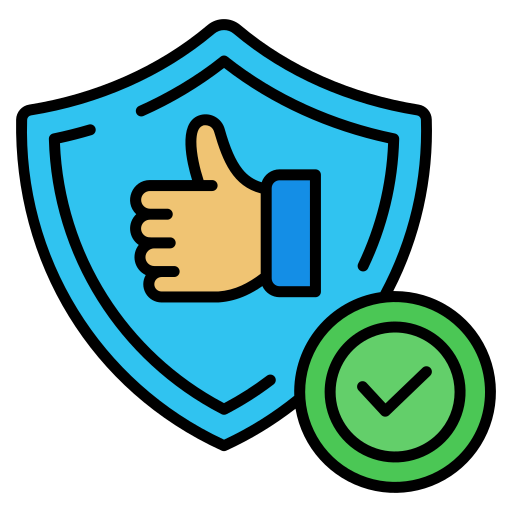
Featured Posts
-
Battle Riot Vii Bobby Fish Among Announced Participants
May 02, 2025 -
Ncaa Basketball Colorado Faces Texas Tech Post Toppins 21 Point Game
May 02, 2025 -
Huntington Whiteleys All White Lingerie Look Simple Elegance
May 02, 2025 -
The Story Of Pancake Day From Shrove Tuesday To Modern Celebrations
May 02, 2025 -
Christina Aguileras Transformation Analysis Of Her Recent Look
May 02, 2025
Latest Posts
-
5 Effective Ways To Foster Mental Health Acceptance In Your Community
May 03, 2025 -
800 Emergency Calls Tulsa Firefighters Confront Winter Weather Challenges
May 03, 2025 -
Winter Storm Overwhelms Tulsa Firefighters 800 House Fires And Rescues
May 03, 2025 -
Tulsa Fire Department Battles Winter Storm Over 800 Emergency Calls
May 03, 2025 -
Tulsa Prepares For Winter Storm Road Pre Treatment In Progress
May 03, 2025