AI's Cognitive Abilities: An Assessment Of Current Limitations
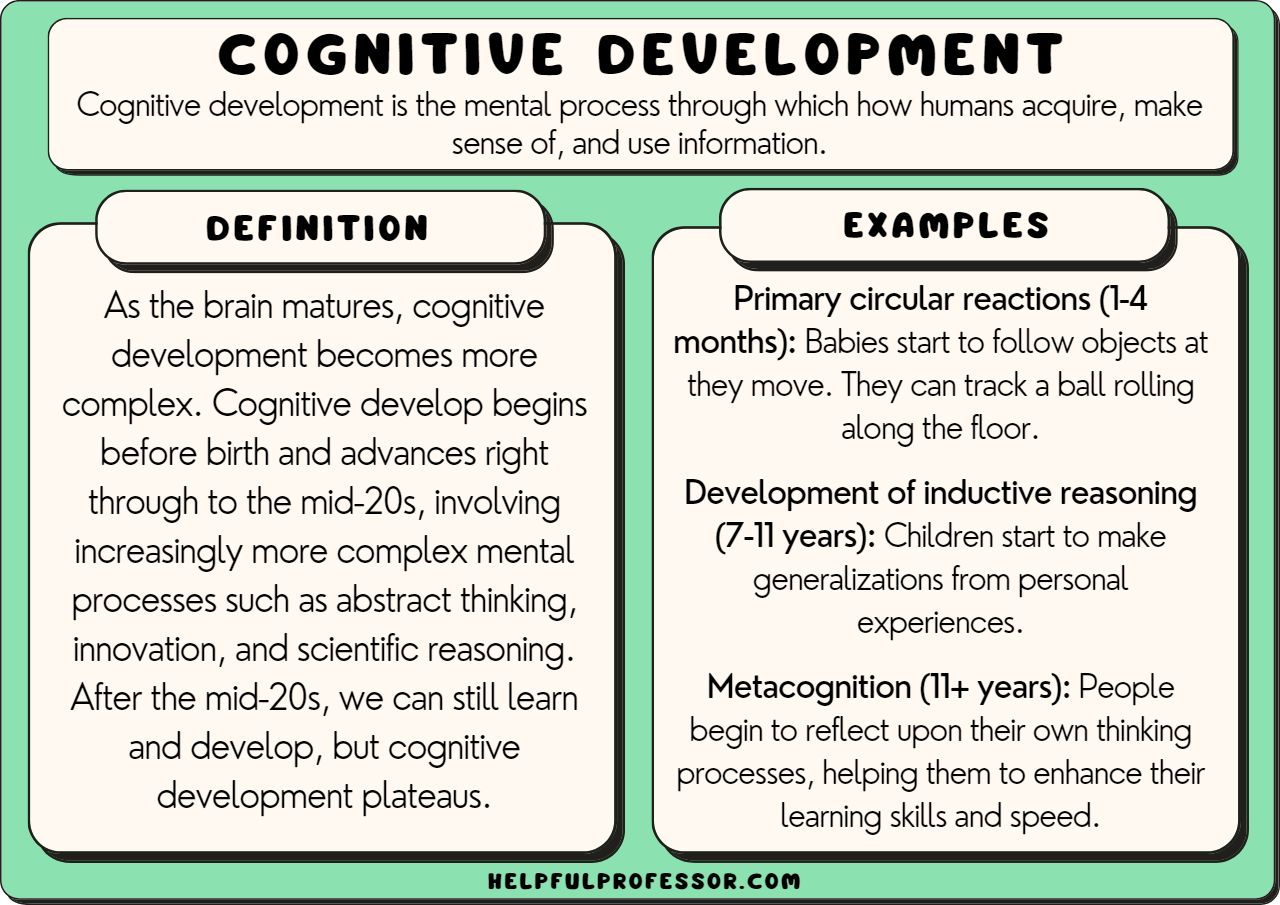
Table of Contents
Artificial intelligence (AI) has made remarkable strides, showcasing impressive cognitive abilities in various domains. From image recognition to natural language processing, AI systems are increasingly adept at tasks once considered exclusively human. However, a critical assessment reveals significant limitations in AI's cognitive capabilities. This article explores these current limitations, examining the gaps between human intelligence and the current state of AI, focusing on the need for further development to unlock the true potential of this transformative technology.
Lack of Common Sense and Real-World Understanding
AI's cognitive abilities often falter when confronted with situations requiring common sense reasoning and real-world understanding. This gap represents a major hurdle in achieving truly intelligent systems.
Contextual Awareness
AI struggles with the nuanced context inherent in human communication and decision-making. This lack of contextual awareness leads to frequent misinterpretations and illogical conclusions.
- Difficulty in understanding sarcasm, humor, and subtle social cues: AI often interprets these elements literally, leading to humorous, but also problematic, misunderstandings. The subtleties of human language, including intonation and body language (missing in text-based interactions), are lost on many current AI models.
- Inability to generalize knowledge learned in one context to another: An AI trained to identify cats in one image dataset might fail to recognize a cat in a different setting, even if the cat is clearly visible. This lack of transfer learning highlights the limitations of current AI approaches.
- Limited understanding of real-world physics and common sense reasoning: AI might struggle with simple tasks such as understanding why an object falls to the ground or predicting the outcome of simple physical interactions. This limitation arises from the lack of embodied experience and intuitive understanding of the physical world.
For example, an AI might misinterpret the sentence "I love this weather" sarcastically spoken during a blizzard. The system would need a deep understanding of contextual cues and social dynamics to correctly interpret the speaker's intent, a feat currently beyond the abilities of most AI systems. Building AI systems capable of this level of reasoning is a significant challenge in the field of cognitive computing.
Embodied Cognition and Physical Interaction
The lack of a physical body significantly limits AI's ability to interact with the physical world in a truly intuitive way. This is a critical aspect of human cognition that is currently lacking in most AI systems.
- Difficulty in navigating complex environments: While robots can navigate structured environments, they often struggle in unstructured or dynamic settings, like a crowded room or an uneven terrain. Human-like dexterity and adaptability are lacking.
- Limited ability to manipulate objects dexterously: Picking up a delicate object or performing precise manipulations are challenges for current robotic systems. Human hands possess a level of dexterity and fine motor control that is yet to be replicated in AI.
- Inability to learn through physical experiences: Humans learn a great deal through direct physical interaction with the world. This embodied learning process is currently largely absent in AI systems.
The development of truly robust robots requires substantial advancements in areas such as sensorimotor integration, real-time control, and the incorporation of learning from physical experiences. This area of research is crucial for bridging the gap between AI's current capabilities and the level of physical interaction found in human intelligence.
Data Dependency and Bias
AI systems are highly reliant on large amounts of data for training. This reliance introduces inherent vulnerabilities, including bias and limited generalizability.
Data Bias and Fairness
AI models trained on biased data can perpetuate and even amplify existing societal biases. This is a serious ethical concern with significant implications.
- Examples of biased AI systems in areas like facial recognition and loan applications: Studies have shown that facial recognition systems often perform poorly on certain demographics, and loan applications can be unfairly denied based on biased algorithms.
- The difficulty in ensuring fairness and eliminating bias in AI algorithms: Addressing bias requires careful data curation, algorithmic design, and ongoing monitoring.
- The need for diverse and representative datasets: Ensuring fairness necessitates training AI on data that accurately represents the diversity of the population.
The ethical implications of biased AI are far-reaching, and addressing this issue requires interdisciplinary collaboration between AI researchers, ethicists, and policymakers. The development of fairness-aware algorithms and methods for detecting and mitigating bias in data are crucial steps towards creating more equitable AI systems.
Data Scarcity and Generalization
Lack of sufficient data for specific tasks hinders AI's ability to generalize and adapt to new situations. This is particularly challenging for specialized domains or rare events.
- Challenges in training AI for rare events or specialized domains: The scarcity of data makes it difficult to train AI models for tasks such as medical diagnosis or fraud detection where data is limited.
- The difficulty in transferring knowledge learned from one dataset to another: AI models often struggle to generalize knowledge learned in one context to another, limiting their adaptability.
- The need for more efficient learning methods: Developing more efficient learning methods that can work with limited data is crucial for improving AI's generalizability.
This limitation necessitates the exploration of alternative learning paradigms, such as unsupervised and reinforcement learning, which can help AI systems learn from less structured data. The development of more efficient and robust learning algorithms is a key area for future research in AI.
Explainability and Transparency ("The Black Box Problem")
Understanding how AI systems reach their conclusions remains a significant challenge, often referred to as the "black box" problem.
Interpretability and Trust
The lack of transparency in many AI models makes it difficult to trust their decisions, especially in high-stakes applications.
- Challenges in explaining complex neural network decisions: The intricate nature of deep learning models makes it difficult to understand the reasoning behind their predictions.
- The need for explainable AI (XAI) techniques: Developing techniques to make AI systems more transparent and interpretable is crucial for building trust.
- The importance of building trust in AI systems: Without transparency and explainability, it will be difficult to widely adopt AI in critical sectors like healthcare and finance.
The opaque nature of many current AI systems hinders their acceptance and deployment in sensitive applications. XAI aims to address this by providing insights into the internal workings of AI models, thereby enhancing trust and accountability. However, developing truly effective XAI techniques for complex models remains a major challenge.
Debugging and Error Detection
Identifying and correcting errors in AI systems is challenging due to their complexity.
- Difficulty in pinpointing the source of errors in deep learning models: The intricate architecture of deep learning models makes debugging difficult and time-consuming.
- The need for robust error detection and correction mechanisms: Developing methods for automatically detecting and correcting errors is crucial for improving the reliability of AI systems.
- The role of human-in-the-loop systems: Incorporating human oversight and intervention can help mitigate errors and improve the overall performance of AI systems.
Robust error detection and correction mechanisms are critical for ensuring the reliability and safety of AI systems, particularly in high-stakes applications. Further research into methods for debugging and validating AI models is vital.
Conclusion
While AI's cognitive abilities have progressed significantly, considerable limitations remain. Addressing the challenges of common sense reasoning, data bias, explainability, and generalizability is crucial for realizing the full potential of AI. Further research and development in areas like embodied cognition, robust learning algorithms, and explainable AI (XAI) are essential. Overcoming these limitations will not only improve AI performance but also ensure its responsible and beneficial deployment. Continued investigation into AI's cognitive abilities is vital for navigating the ethical and practical considerations of this transformative technology. The future of AI hinges on overcoming these limitations and building systems that are not only powerful but also trustworthy and beneficial to humanity.
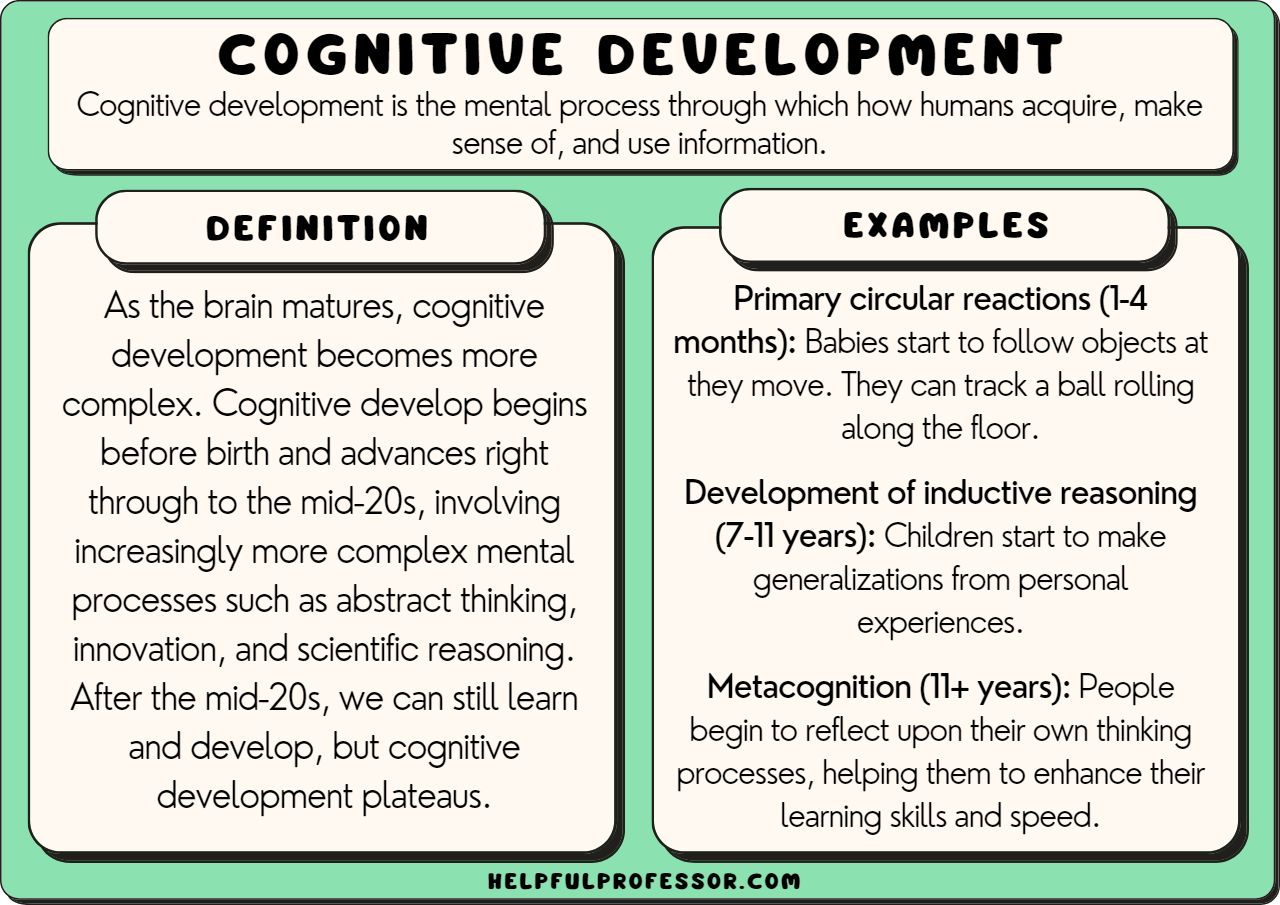
Featured Posts
-
Beyond Bmw And Porsche The Broader Automotive Struggle In The Chinese Market
Apr 29, 2025 -
Teens Rock Throwing Leads To Murder Conviction
Apr 29, 2025 -
See Bob Dylan And Billy Strings Live Portland Outlaw Music Festival
Apr 29, 2025 -
Vincenzo Grifo Ciro Immobile Luca Toni Andrea Barzagli Ruggiero Rizzitelli Italian Stars Who Conquered The Bundesliga
Apr 29, 2025 -
Ray Epps V Fox News Examining The Defamation Claim Related To January 6th
Apr 29, 2025